How will Next Generation's Products be Developed & Deployed?
- Plato Pathrose
- Oct 5, 2024
- 6 min read
Tomorrow comes with a lot of surprises. It will be driven mainly by Artificial Intelligence (AI) in the technology world. Applying AI in different industries and areas will bring multiple opportunities in the product and service sectors. In this article, we will discuss some of the possible applications of AI and how this can benefit product development. The proposed applications and possibilities are not specific to any specific industry; instead, they can be applied across different industries such as Automotive, Banking, Industrial Automation, Healthcare, etc. For a better understanding and to keep the technical depth in the article, we will go through the application and the usage of AI in product development and deployment, navigating through the different lifecycle phases in systems engineering processes as a reference.
One of the mainstream uses of AI these days is to generate data. This was made feasible by introducing Generative AI, or Gen AI. Generative AI is an artificial intelligence technology that can produce various types of content, including text, imagery, audio, and synthetic data. Gen AI became powerful with the introduction of GANs a decade back, which opened up many opportunities for its usage and application until today. Along with GANs, two other notable introductions from the machine learning stream have made drastic evolutions in the application of AI. They are Transformers and Large Language Models or simply LLMs. Transformers help train the larger models without labeling all the data in advance. As they can also establish connections between various data points through the notion called ‘attention,’ the results in their output become rich and reliable.
The advancement of LLMs has opened a new era in which Gen AI models can write engaging texts, generate videos, photo-realistic images, etc., that can be used for various purposes. As we have moved from classical product development towards data-driven product development, data generation, and usage have become increasingly important. Data generation and availability can be cleverly used across various phases of product development.
Many organizations have initiated digitalization and introduced Gen AI in their organizational ecosystem, connecting various stakeholders. One common mistake in digitalization and Gen AI applications is applying Gen AI without enough analysis of its utilization and benefits. Many organizations tried to deploy it by completely replacing the majority of their existing toolchain, which cost them a fortune, and the benefit they got is negligible compared to the costs spent on these tool revisions. One of the critical areas many would miss in their studies during the digitalization approach is the existing processes. Many try to keep the processes as they are when they try to digitalize and introduce the benefits of AI without knowing why such processes exist. Some of those processes might not even be relevant to digitalization and the usage of AI. One should identify those and tailor them as required to optimize the deployment. Two facts must be remembered when such digitalization projects are planned.
· Tools are to make our jobs easy and not to make them complex.
· The need for the process is to reduce wastage.
These two should be the foundation for introducing tools and applying Artificial Intelligence models to make the work easier as part of the organizational ecosystem.
AI can act as an enabler for product development and bring many advantages if it is integrated well and optimized in any organization. The IPO diagram below shows an integrated process model for product development based on systems engineering processes. Artificial intelligence, along with organizational infrastructure, tools, and knowledge management systems, can be included as an enabler.
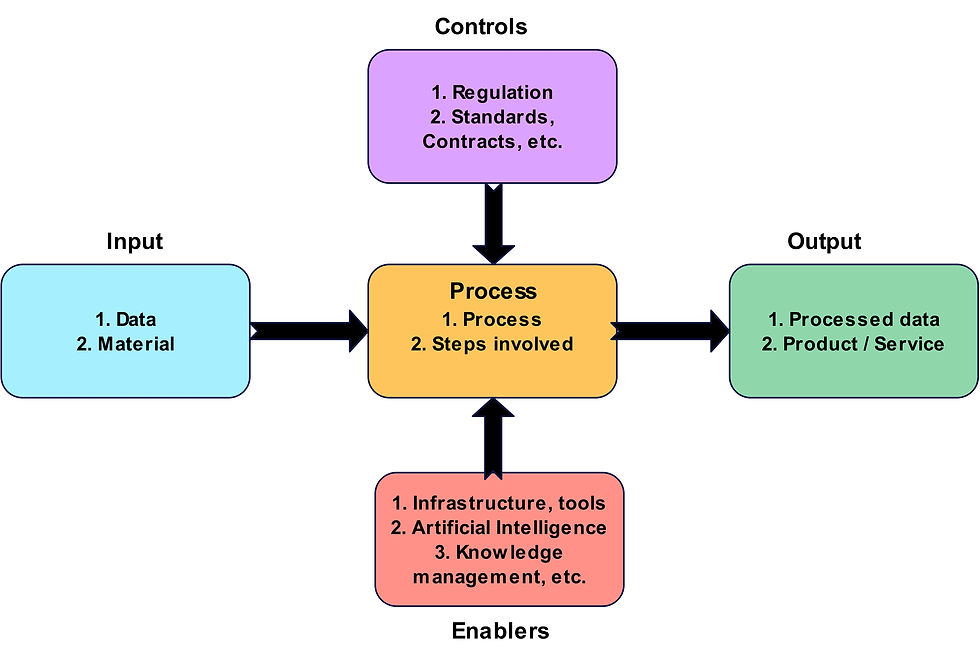
There can be multiple ways to introduce an AI framework in an organization. Taking the example of product development and deployment below, you can see how a Gen AI framework is applied across different product lifecycle phases. Integration of various tools in the organization is the key to having the advantage of any such application. The integration with Gen AI models such as LLMs or transformers can be made at any process associated with the product's lifecycle. As long as the tools are not integrated and are operating in tandem, the benefits might not be visible, and we cannot claim that the application of AI improved something or brought any advantage to the overall ecosystem.
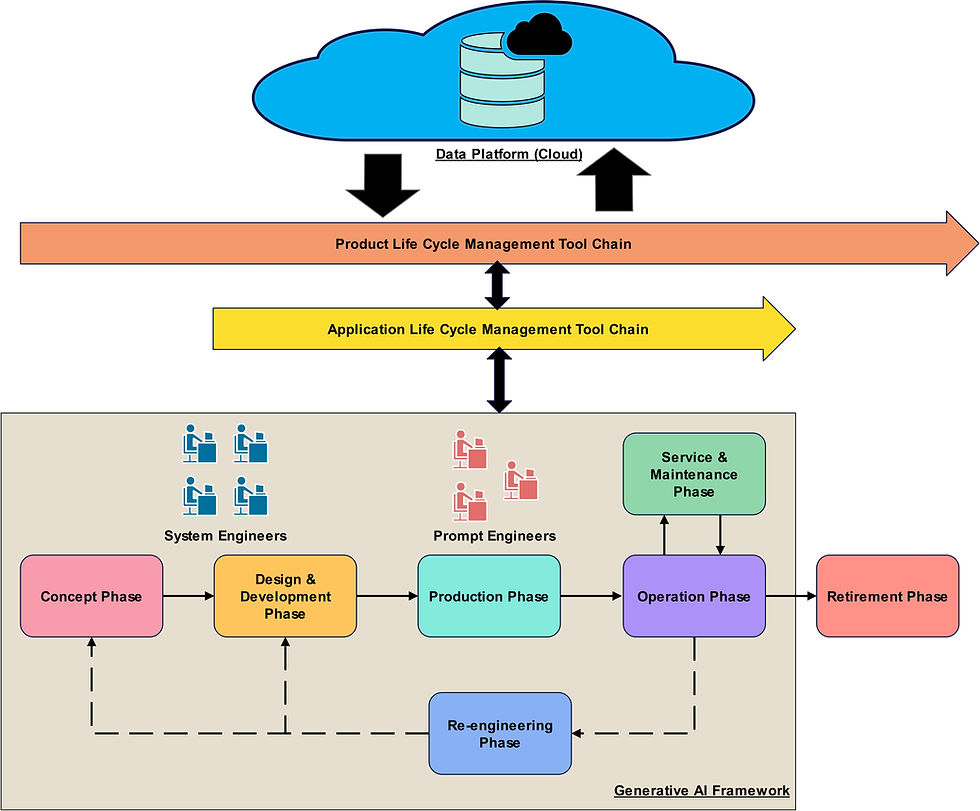
The high-level architecture above integrates various product life cycle phases with the organizational toolchains for Application Lifecycle Management (ALM) and Product Lifecycle Management (PLM) together with the data platform and the Gen AI framework. However, many organizations still lack integration between the ALM and PLM toolchains, which is a challenge regarding data consistency and accuracy over the entire product lifecycle and increases data management complexity.
Introducing Gen AI tools and models in the product development ecosystem brings specific changes in roles or new jobs into the limelight. Prompt engineering is one of those. Prompt engineering is the process of structuring instruction in natural language that can be interpreted and understood by a generative AI model. A prompt is the natural language text describing the task that an AI model should perform and generate results. The quality of the prompt decides the quality of the output, and there will be a demand for prompt engineers soon in the market on a larger scale. Prompt engineers must work together with the system engineers in product development during its different lifecycle phases. Utilizing a large language model (LLM), prompt engineers can generate various use cases and even the possible delighter elements for the product early in the concept phase of the product. These use cases can also be adopted from different industries as well. It is the same as nowadays having touch screens inside vehicles, which was adopted from mobile phones from the consumer industry.
Further integrating the tools with Gen AI models in the product development ecosystem, the generated requirements can be modeled to various models as required if following model-based systems engineering. It can be further decomposed into hardware, software, and mechanical requirements. Using Gen AI models, corresponding test cases can be generated using prompts based on these requirements. With the strength of specific language models these days, it is possible to translate those generated test cases into lines of code for test automation. If the tests are based on events or scenarios, the Gen AI models help in programming events and scenarios with various tools used for simulation. The iterative approach of development these days using agile methodologies can work well with digitalization and Gen AI integration into the operational ecosystem for product development. Integrating Gen AI models with the DevOps and Testing tools can save time and effort if the input and generated data are reliable and controlled. This requires robust pre-deployment tests and qualification.
Moving to the production phase, the integration and tests for the sub-assemblies and assemblies can be automated with the reliable generation of steps and tests after integration. Deploying embedded AI models into the system and deploying them in the market can bring certain advantages, such as predictive maintenance and enhanced product life. This will increase operational time, enhance safety, prolong life, and, most importantly, customer satisfaction.
The re-engineering phase, which is not usually part of the standard systems engineering lifecycle, is also included here. This is the optional phase through which many products go once they are deployed in the market. This phase can be for various reasons, such as re-engineering for cost optimization, integrating new functionalities, integrating requirements due to regulatory changes, market expansions, or fixing bugs. Gen AI models with the tools can help the re-engineering phase by incorporating new requirements and test cases and identifying possible replacement options for optimization initiatives, such as replacing an SoC (System on Chip) or microcontroller or specific other components that fit into the overall system.
Digitalization and the integration of AI provide more significant advantages and power. The technology ecosystem will experience a drastic change in the next few years as these next-generation technologies are applied, and they act as enablers in any product development. As we see we will have great power with these technologies, we must also be cautious. ‘With great power comes great responsibility’. We should be cautious and accept that drawbacks and possible threats come with these advantages. The importance of cybersecurity and safety should not be left behind when we try to bring in technological advancements. Since many AI components are already integrated and deployed in the products, bringing AI models as part of tools and infrastructure will also add risks and challenges. The European Union AI Act from 2023 classifies the systems as low, medium, and high based on their associated risk. Automotive systems that provide autonomous functionalities and medical systems come under high-risk systems. Hence, specific processes, assessments, and audits must be established and evaluated to deploy those systems in the EU market.
*This is an excerpt from my new book on Next Generation Product Development and Deployment
Comments